You are currently visiting the test version of the radboud Dspace repository. To access the production instance, you can navigate to: https://repository.ubn.ru.nl
Memory-efficient 2.5D convolutional transformer networks for multi-modal deformable registration with weak label supervision applied to whole-heart CT and MRI scans
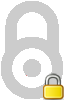
Fulltext:
215834.pdf
Embargo:
until further notice
Size:
3.023Mb
Format:
PDF
Description:
Publisher’s version
Publication year
2019Source
International Journal of Computer Assisted Radiology and Surgery, 14, 11, (2019), pp. 1901-1912ISSN
Publication type
Article / Letter to editor

Display more detailsDisplay less details
Organization
Medical Imaging
Journal title
International Journal of Computer Assisted Radiology and Surgery
Volume
vol. 14
Issue
iss. 11
Page start
p. 1901
Page end
p. 1912
Subject
Radboudumc 9: Rare cancers RIHS: Radboud Institute for Health Sciences; Medical Imaging - Radboud University Medical CenterAbstract
PURPOSE : Despite its potential for improvements through supervision, deep learning-based registration approaches are difficult to train for large deformations in 3D scans due to excessive memory requirements. METHODS : We propose a new 2.5D convolutional transformer architecture that enables us to learn a memory-efficient weakly supervised deep learning model for multi-modal image registration. Furthermore, we firstly integrate a volume change control term into the loss function of a deep learning-based registration method to penalize occurring foldings inside the deformation field. RESULTS : Our approach succeeds at learning large deformations across multi-modal images. We evaluate our approach on 100 pair-wise registrations of CT and MRI whole-heart scans and demonstrate considerably higher Dice Scores (of 0.74) compared to a state-of-the-art unsupervised discrete registration framework (deeds with Dice of 0.71). CONCLUSION : Our proposed memory-efficient registration method performs better than state-of-the-art conventional registration methods. By using a volume change control term in the loss function, the number of occurring foldings can be considerably reduced on new registration cases.
This item appears in the following Collection(s)
- Academic publications [244578]
- Electronic publications [132441]
- Faculty of Medical Sciences [92890]
Upload full text
Use your RU or RadboudUMC credentials to log in with SURFconext to upload a file for processing by the repository team.